On the Way to Lakehouse
Table of contents
According to a study from Databricks and Fivetran, 65% of surveyed organizations have already implemented a data lakehouse for their analytics needs, with another 42% of those who haven’t done it planning to adopt this data management model over the next 12-24 months. Should you jump on the bandwagon, or are there other efficient data management solutions for your business? We are going to explain how existing data architectures fit into the needs of different industries and illustrate them with cases implemented by the IBA Group team.
How Business Needs and New Technology Drive Data Platforms Evolution
A good while ago, in the mid-1960s, when data was stored in files providing strictly sequential access, Charles Bachman came up with the idea that it would be much easier to find and use data if a programmer could specify a way to the information they needed. This led to the emergence of first-generation navigational databases represented by network and hierarchical models. Although ensuring rapid data access, navigational data storage solutions suffered from rigorous data structure requirements, which encouraged Edgar Codd from IBM to invent the relational model for database management in the early 1970s.
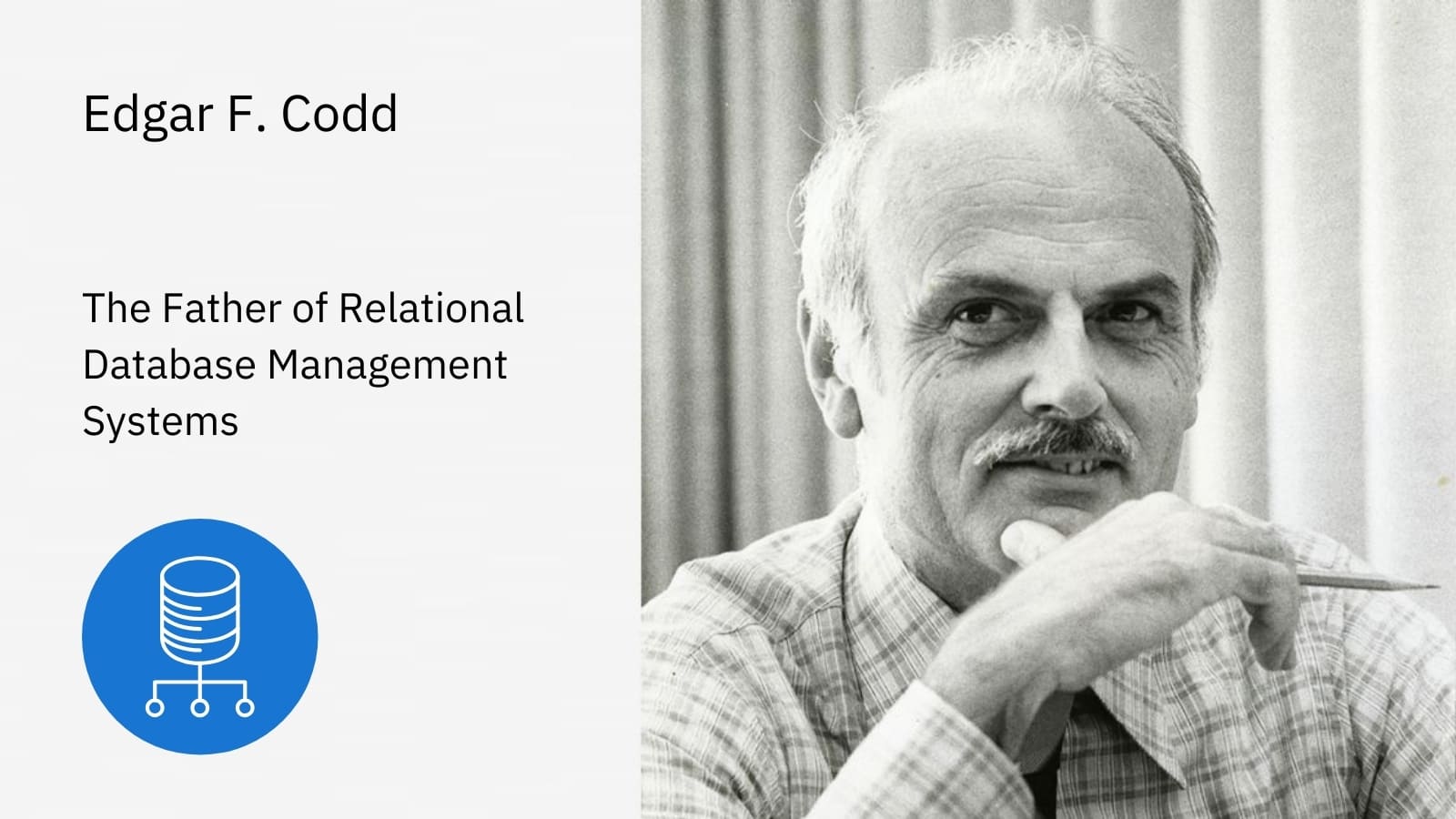
The Advent of the Data Warehouse
With the introduction of relational databases and the growth of data amounts generated by business applications, the next concept loomed on the horizon — data warehouses. Businesses wanted to use information collected through their operational systems in decision-making and needed centralized data management solutions to incorporate data from multiple sources and handle complex analytical queries. By the 1990s, organizations had enjoyed a choice from two designs for data warehousing solutions:
- the top-down approach based on a subject-oriented normalized structure offered by Bill Inmon;
- the bottom-up architecture based on dimensional modelling developed by Ralph Kimball.
There will definitely still be some space for these types of solutions in the modern world. Synergy Research Group, a US market intelligence and analytics group, predicts that in 2027, on-premises solutions will still have a sizable share of the market.
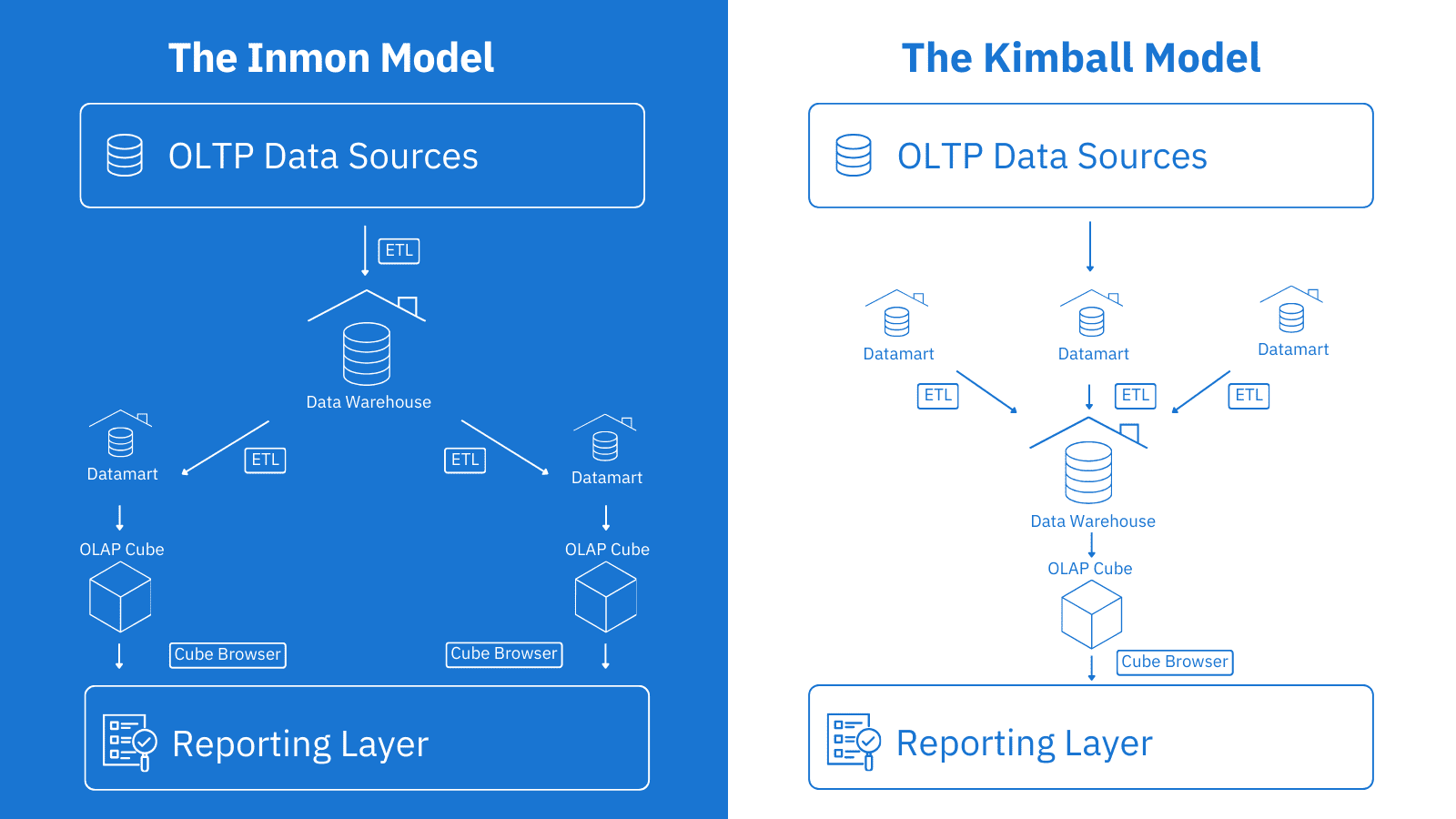
Big Data Gives Rise to Data Lakes
The noughties brought another challenge to the data management landscape — big data. With the explosion of web applications, mobile technology, and social media, companies got the possibility to collect and analyse more information from a greater number of sources, but the increased data volume made it difficult to process and store. While data warehousing solutions were trying to cope with the load by structuring data within their repositories, the new concept of data lakes arrived, stating that data can be stored in its raw form. The idea gained traction with the development of cloud data platforms, which delivered the infrastructure and scalability needed to handle large volumes of data.
The Data Lakehouse Embraces New Technology
In the early 2010s, the first cloud data warehouses appeared, followed by even more advanced solutions like multi-cloud data platforms. Businesses also got access to user-friendly self-service business intelligence tools, which allowed users to access, analyse, and visualize data without the help of IT or data specialists. Over time, both the data lake and data warehouse began integrating with AI since the new technology could help greatly in big data processing. As AI and ML algorithms enhanced enterprise data management, this led to advanced analytics possibilities and even wider BI adoption across organizations.
By the 2020s, the rise of machine learning and real-time data processing had triggered a movement to a more AI-centric big data storage model — the data lakehouse. The hybrid data lakehouse nature brings together the high performance of the structured DWH environment with the flexibility and scalability of data lakes. However, the data lakehouse is also not a one-size-fits-all solution, and a company needs to choose a data architecture depending on the maturity of its data analytics, the type of analytical tasks it faces, and the diversity of data types it works with. Let’s see how different data management solutions can serve business goals in various industries.
DWH as the Foundation of Data Management
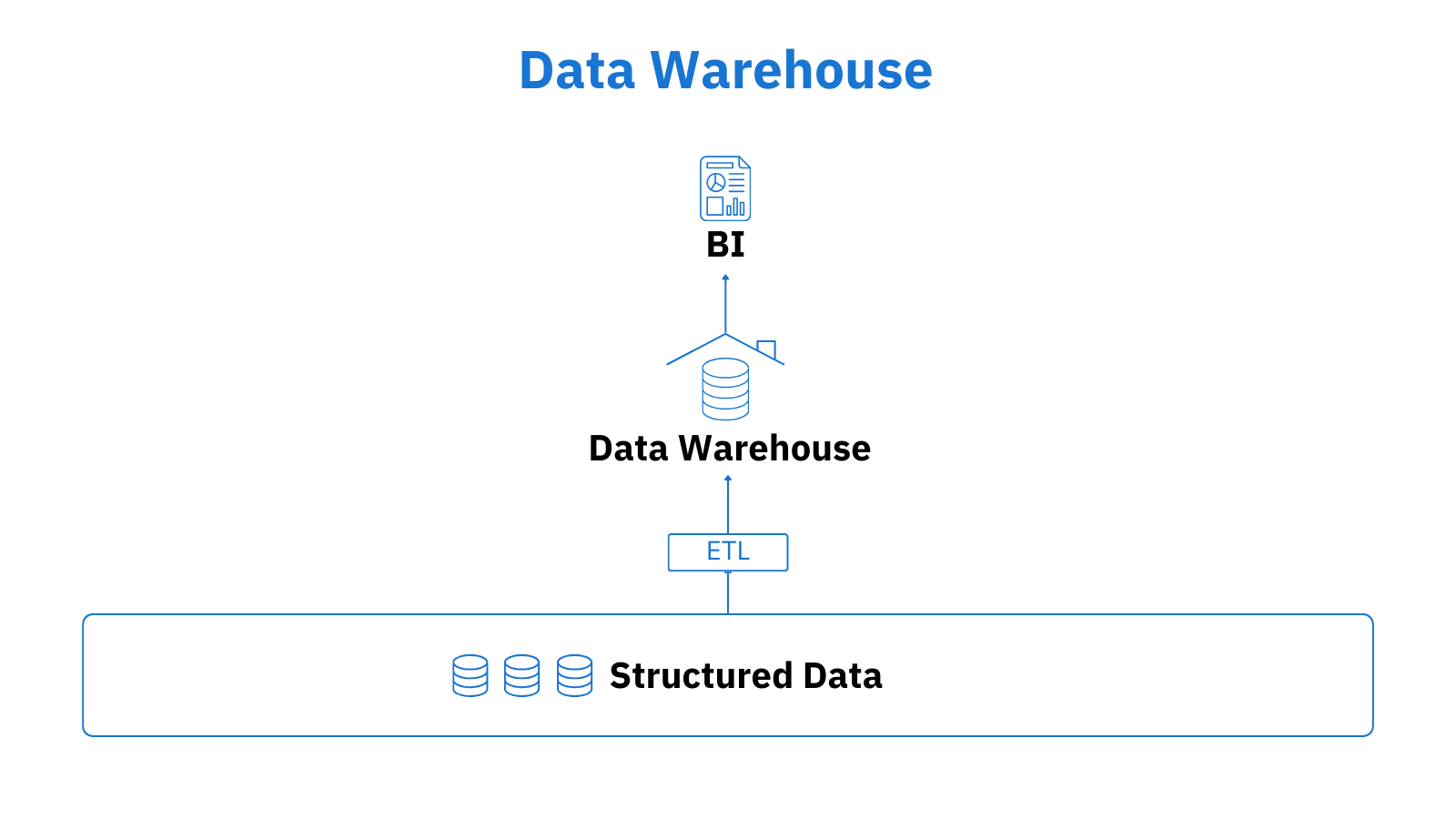
A data warehouse works as a hub processing and consolidating data from numerous sources to generate analytics in response to SQL queries. It arranges the aggregated data into accurate, labelled boxes based on specific schemas, which helps with data quality management and makes user interaction with the data much easier. These data management solutions are designed to better deal with data that is structured in a predefined, standardized way.
But isn’t the data warehouse outdated?
Not at all. Data warehouses have undisputable strong points that keep them relevant and in demand in present-day scenarios. They are integrated, fully managed, and easy to build and operate, which is just what many modern businesses want. A range of established vendors, such as Google BigQuery, Oracle Autonomous Data Warehouse, Snowflake, and Amazon Redshift, offer all-in-one solutions, gathering metadata, storage, and computational power under one roof, thus contributing to simplified enterprise data management.
And how does the data warehouse satisfy modern data management requirements?
Nowadays, we have an updated version of data warehouses, which have perfectly adapted to new realities and ever-changing business needs. Unlike the warehouse of the past, which was costly to deploy and took quite a long time to set up, they evolved into more scalable and flexible cloud data solutions, which require less technical configuration and are available in the more cost-efficient “pay-as-you-go” pricing model. On top of handling big data well, they use ML and AI to deliver advanced analytics for businesses to find insights in data and predict future trends for better decision-making.
When do you need a data warehouse?
While data warehouses are suitable for many industries and scenarios, here are some examples illustrating their real-life application.
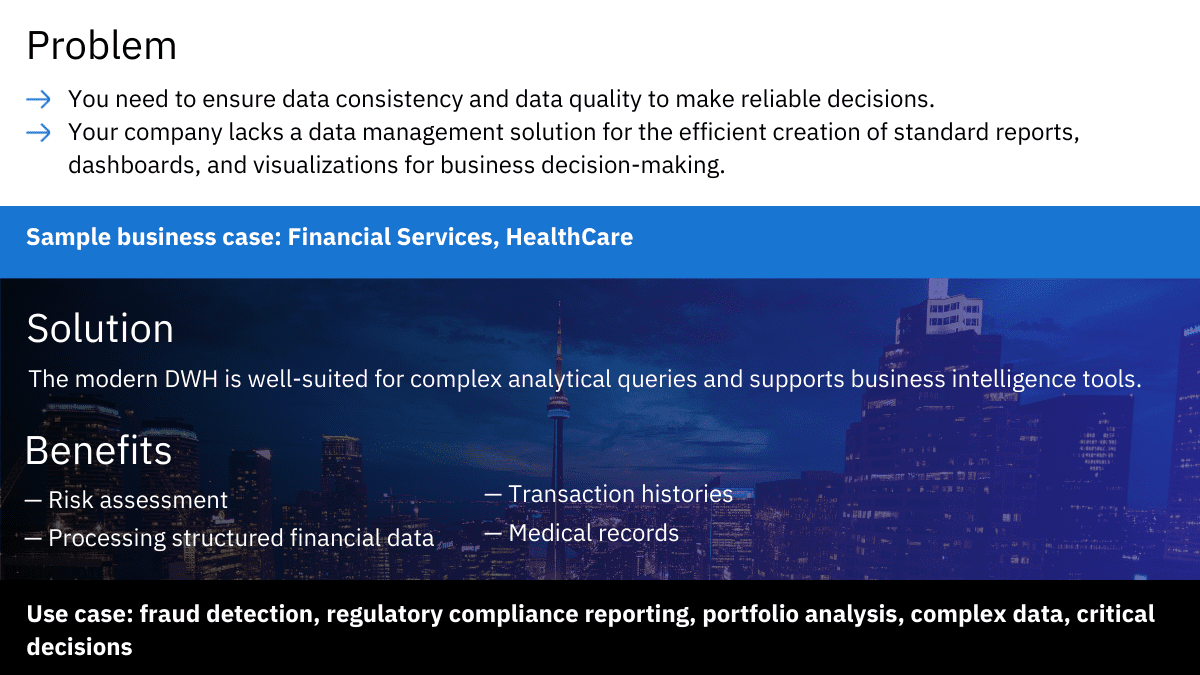
Case study
A bank needed to improve its reporting system based on IBM Cognos and IBM InfoSphere DataStage. To achieve this goal, the data management team of IBA Group developed:
- an Enterprise Information System (EIS);
- an Enterprise Data Warehouse (EDW);
- reports on suspicious transactions;
- compliance reports;
- Market Risk Data Mart;
- processes to retrieve bank customers’ data from the EDW;
- ETL tools for the “Unified Register of Customers” software package;
- ETL processes to back up the archive and separate Operational and Archive analytical models.
Our certified instructors also provided training for the customer’s team along with follow-up support. As a result, all information required for analysis and sourced from different enterprise systems was combined in the EDW and EIS. The bank’s employees can now create more detailed financial reports assisting management in making informed decisions.
Do we need to give preference to the data lake vs the data warehouse?
Despite the current craze over streaming data, businesses still need historical data to track changes in organizational performance, market behaviour, and customer preferences to spot trends and plan for the future. That is what data warehouses are good at, and that is why there is hardly an enterprise that doesn’t have these data management solutions.
While data scientists and self-service data consumers enjoy multiple benefits of the data lake, many BI specialists, business analysts, and other information workers don’t really want to tinker with self-managed data systems. All they need is fast and simple access to a wealth of time-variant relational data, systematically cleansed and perfectly integrated. They surely won’t be happy to swap the warehouse for the data lake or data lakehouse since the tried-and-true solution continues to cater to their needs and bring value.
Data Lake as a True System of Record
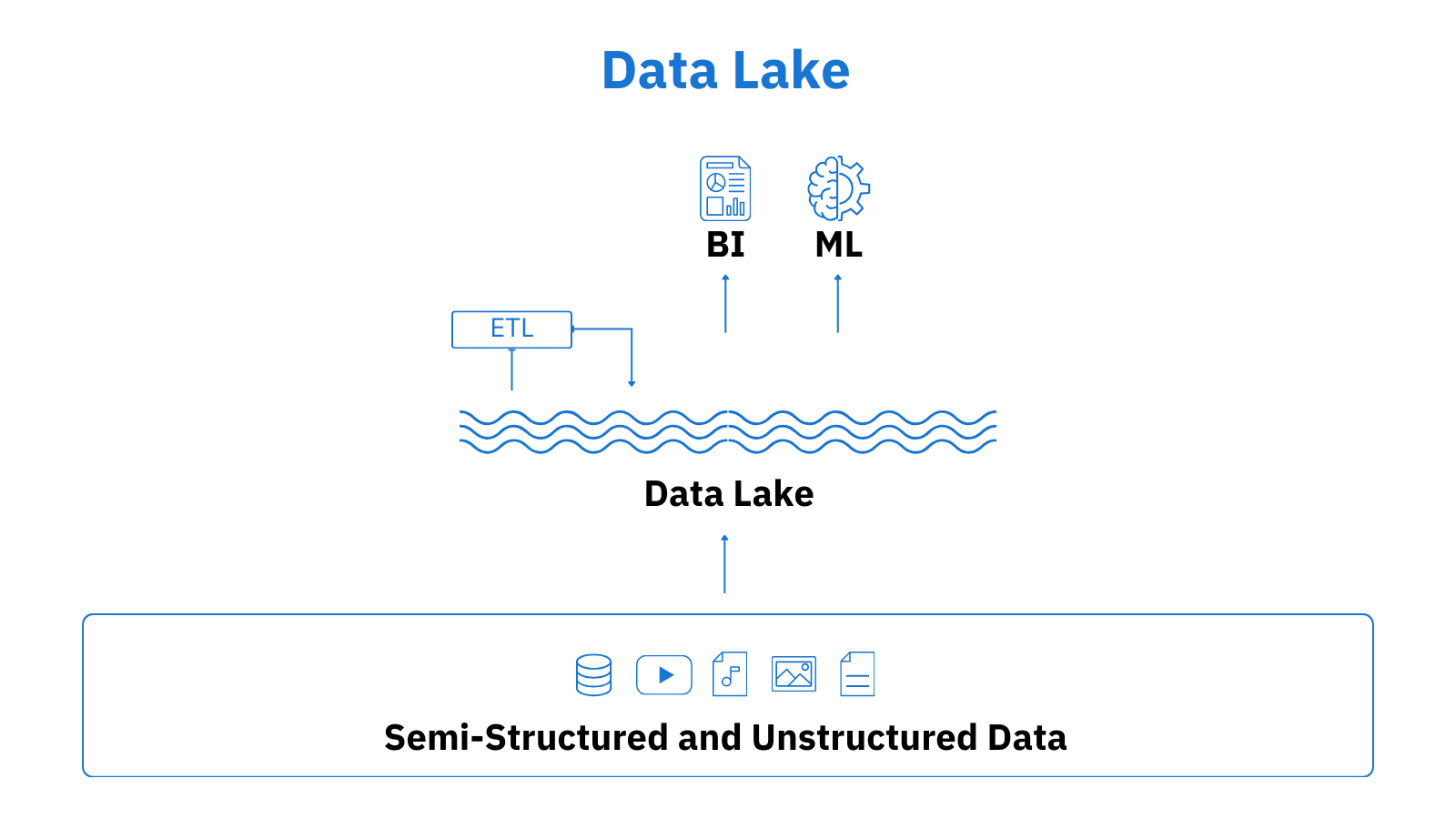
Much like warehouses, data lakes can accommodate a good deal of data coming from different types of sources, yet without any transformations, which turns them into the true system of record. One of the main data lake benefits is that, apart from structured data integration, they can digest various kinds of unstructured or semi-structured data, such as pictures, videos, emails, or even sound recordings. In fact, this data type can make up to 90% of data available to organizations, and it often provides phenomenal value. Delivering a rich collection of data and decoupling storage and computing, data lakes are well-suited for data science, streaming, and machine learning.
What makes data lakes more flexible than data warehouses?
Since data lakes can handle anything from highly structured to loosely assembled data and store it in the raw format, they keep up with diverse analytical needs, including scenarios that require access to data in its most unprocessed form. They boast synchronization with more data science tools and allow data engineers to choose a tech stack based on specific requirements.
Data lakes provide a bulk of unstructured data for data scientists deploying ML models and are best suitable for businesses with data engineers in their teams to ensure support for their customized platforms. However, the emergence of easy-to-use managed solutions has reduced the need for in-house data specialists.
When do you need a data lake?
Here are some of the goals you can achieve by deploying a data lake.
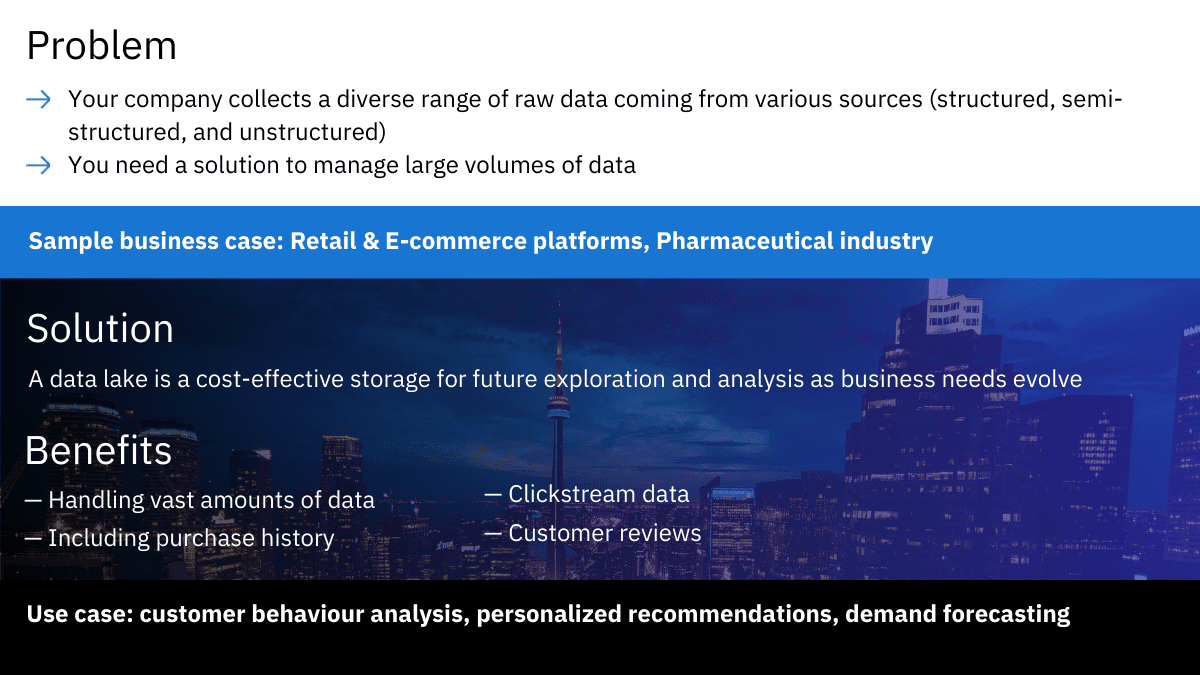
Case study
A pharmaceutical company needed a centralized data management platform to replace its legacy data storage solutions with the data scattered across systems and, thus, failing to provide a unified view. To help the client get control of its data, IBA Group:
- created a data lake for CRM and merchandising purposes;
- implemented data processing techniques and methodologies to measure KPIs;
- redesigned dashboards displaying sales activity, product visibility, and distribution;
- performed integration into a global DWH solution;
- ensured compliance with stringent pharmaceutical regulations;
- streamlined data processes.
Thanks to the cloud data lake implemented by IBA Group, the company improved product visibility and placement, automated and enhanced control of merchandising activities, got the opportunity to receive x2 quicker insights from clinical trials, and benefited from significant infrastructure and manpower cost savings.
Why do data lakes degrade to data swamps?
If designed and implemented correctly, data lakes don’t replace your data warehouses but complement them. However, putting these things together to work efficiently definitely requires some skill. Without proper design, chances are that the data lakes’ unstructured nature will result in a performance decrease while the limitations in parallel querying can create bottlenecks. Better suited for writes than for reads, data lakes require well-defined governance, and if this is not the case, they easily turn into data swamps. The mess of data leads to failed data discovery and produces little analytical insights.
Data Lakehouse as an Innovative Data Architecture
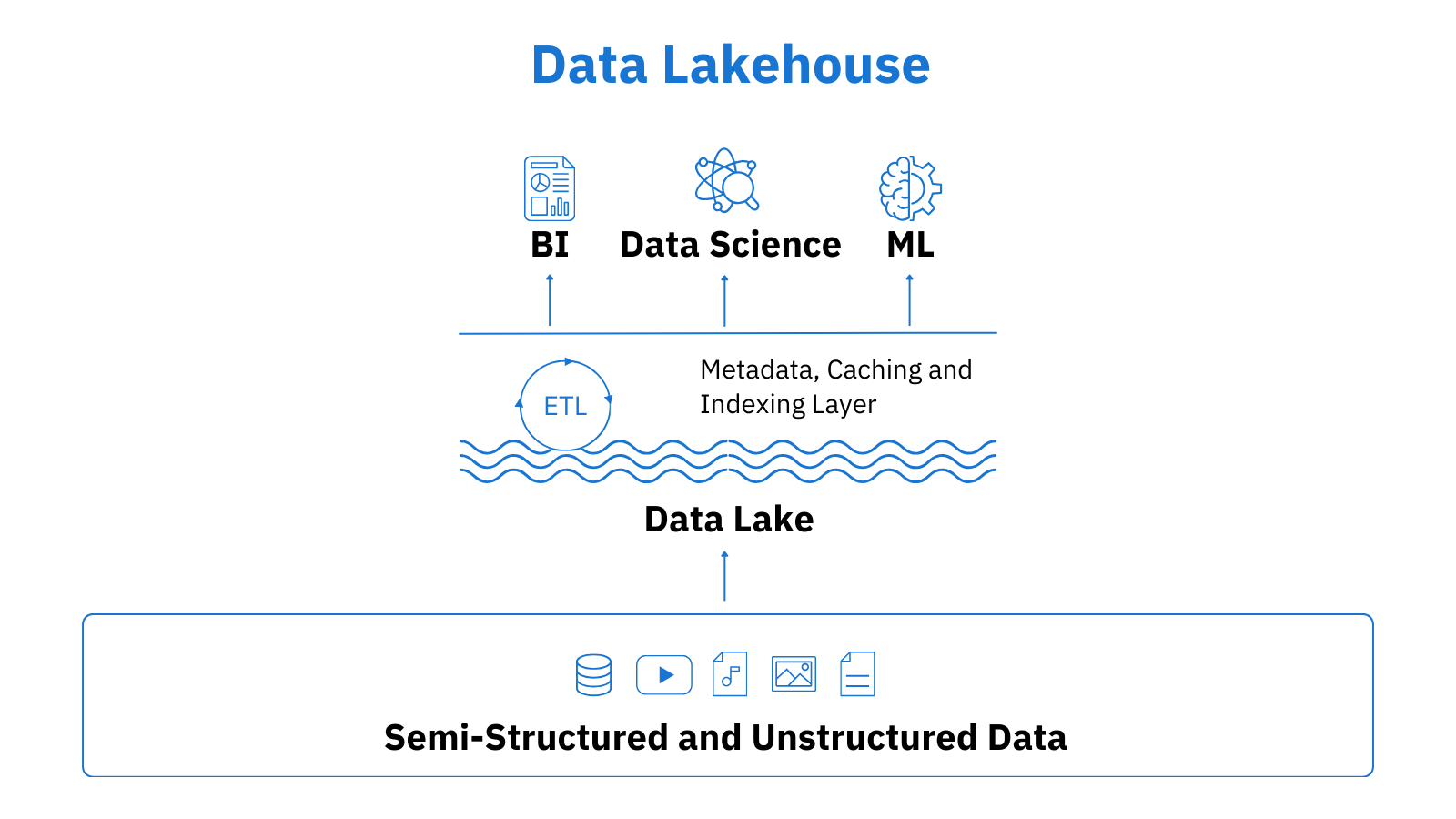
The data lakehouse arrival was driven by cloud providers wishing to make their warehouses more flexible and expand their analytical capabilities. They started to add some features of the data lake to data warehouse solutions, for example, support for Apache Parquet, ORC, and other open formats. Vice versa, software engineers found a way to insert some of the warehouse functionality, such as SQL querying and schema definitions, into data lakes for higher performance and improved governance. The combined efforts culminated in an optimized data architecture now known as the data lakehouse.
How does the data lakehouse serve businesses with diverse data needs?
The hybrid architecture of the data lakehouse combines the best features of its predecessors, providing a single repository for structured, semi-structured, and unprocessed data for all teams and departments of an organization, thus eliminating data silos. By employing Presto and Spark technologies, it ensures real-time data processing for immediate information delivery while also supporting batch processing for large-scale analysis and reporting. With a metadata and governance layer deployed over the lake architecture, the data lakehouse can go beyond ML and data science scenarios, serving BI and reporting needs directly, without prior summarization in data warehouses.
Besides, cloud providers offer managed data lakehouse services nowadays, relieving data consumers from the need to sweat over self-service solutions. With tools like Apache Hive, Delta Lake, and Apache Spark by Databricks or Amazon’s Athena and Glue, businesses can build and manage their hybrid data management platforms in a much easier way.
When do you need a data lakehouse?
Thanks to the combined power of the lake and warehouse concepts, data lakehouses boast diverse applications, with only some of them illustrated below.
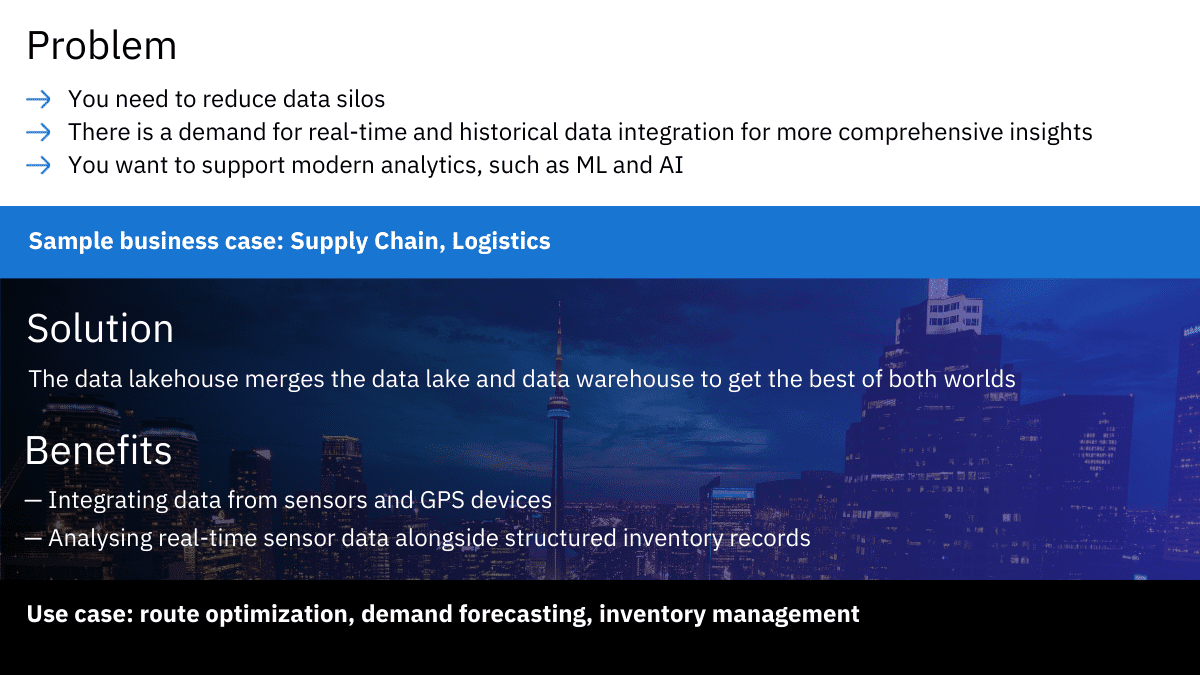
Case study
A large global IT company needed to transfer its client’s infrastructure, which was based on the Oracle database and the Informatica Power Center ETL tool, to cloud services. The infrastructure included many data storage systems covering production cost tracking, dialer information, clients’ and employees’ data, and more.
When trying to modernize the entire infrastructure, the end client suffered from the slowdown of their existing services, partial data losses, permanent delays in providing new services, and other workflow bottlenecks. Besides, the framework of the old infrastructure made scaling costly because of the low flexibility of vendor licensing offers. Due to the huge variety of technologies used, the client also faced difficulties with hiring developers having the required skills.
To help the client move company services to new cloud infrastructure, leverage the modern data lakehouse architecture, and tailor AWS vendor migration offerings to the services, IBA Group:
- provided engineers and architects to create custom teams responsible for adaptation and improvement in each area having its own architecture;
- participated in developing the top-level structure of the entire ecosystem;
- took part in defining general approaches and rules for each system;
- engaged in existing services transition to cloud technologies — AWS Glue, ECS, Kubernetes, EMR + PySpark;
- ensured uninterrupted access to existing services during the migration.
After the AWS cloud migration and data lakehouse implementation were completed, the client gained the following benefits:
- increased fault tolerance with fixed bugs and ready-made cloud solutions for interaction between different customer services;
- cost reduction by cutting down on some server capacities;
- flexible financial expense planning for scaling provided by the pay-as-you-go system;
- reduced IT recruiting time with unified requirements, clear rules, and a well-defined technology stack;
- optimized IT teams with narrowly focused specialists retrained in the technology stack adopted by the company.
Building the data lakehouse also enabled the company to better control user access to data and its sharing, analyse a broad range of data types, derive valuable insights from real-time reporting, and improve operation efficiency.
How can data lakehouses ensure data quality?
To prevent a data lakehouse from morphing into a data swamp because of absorbing everything and anything without proper data preparation, a medallion architecture comes into play, enhancing both data quality and structure. It consists of a series of data layers designed to process and logically organize incoming data as it passes through a data lakehouse:
- The bronze layer stores unprocessed data.
- The silver layer provides a refined structure with a validated, enriched version of the data.
- The gold layer contains clean data fully structured for business use.
By passing data through multiple steps of validations and transformations, this data architecture ensures all the ACID properties required for data validity and delivers a well-governed holistic view of enterprise data.
Why do the data lakehouse streaming capabilities give an edge to modern businesses?
Modern apps, transactional systems, activity logs, and Internet of Things (IoT) devices produce a continuous flow of precious data that can be used by businesses for real-time analytics. Data lakehouse platforms capable of processing this streaming data generate a wealth of near real-time insights, helping organizations to faster respond to various events, for example, customer actions, security breaches, changes in market prices, and equipment malfunctions. The shortened reaction times allow businesses to increase customer satisfaction, mitigate adverse events, reduce expenses, and become more competitive overall.
Does the data lakehouse model really suffer from a lack of vendors?
Although the data lakehouse concept is a newcomer to data management, its benefits are so undeniable that it took the analytics world by storm, urging data platforms to address the demand. Databrics, which was named the best data lakehouse vendor of 2024, pioneered the movement by adopting the “data lakehouse” term to market its data intelligence platform. However, other cloud data warehouse vendors, such as AWS and Snowflake, were also moving in the same direction in the early 2020s, although not always labelling their projects as a data lakehouse.
Nowadays, data lakehouse solutions are provided by:
- Databricks
- Snowflake
- Microsoft Azure Synapse Analytics
- AWS
- Teradata VantageCloud
- IBM watsonx.data
- Dremio
- Cloudera Data Platform
- Google BigLake
- Oracle Cloud Infrastructure
The impressive list of vendors proves the abundance of existing data lakehouse solutions and the concepts’ promising prospects despite its infancy.
Meeting the AI Era Fully Armed
The data lakehouse is a perfect fit for the era of big data, BI, and AI convergence, providing the one and only solution enabling businesses to unify all their data on a single platform for every use case, from AI to ETL. The data lakehouse can store large amounts of data, allow you to process them in a structured, high-performance environment, and lay the groundwork for AI-driven project implementation.
If you don’t know which of the above data management solutions suits you best, IBA Group encourages you to undergo a technical interview for a free micro-assessment within our Modern Data Lake and Warehouse service. Our experts will briefly analyse your existing infrastructure and deliver a recommendation plan revealing current issues and offering possible improvements. We will advise you on how to build a data lakehouse or other suitable solution in the most efficient way and provide you with all the necessary tooling and support. Don’t hesitate to get in touch with us.
YOU MAY ALSO BE INTERESTED IN
- Cloud vs On-premises: What is Better for Business?
- Why Migrating to the Cloud Brings Value
- Data Migration to Cloud: What You Will Get in the End
- Why Migrating to the Cloud Brings Value
- ETL/ELT: What They Are, Why They Matter, and When to Use Standalone ETL/ELT Tools
- Data Literacy: The ABCs of Business Intelligence
- BI Tools Comparison: How to Decide Which Business Intelligence Tool is Right for You
- Unsuccessful examples of BI development. Part I
- Examples of Unsuccessful BI Development. Part II
- BI Implementation Plan
- IBA Group Tableau Special Courses
- Integrating Power BI into E-Commerce: How to Succeed in Rapidly Developing Markets
- Analytics vs. Reporting — Is There a Difference?
- Better Business Intelligence: Bringing Data-Driven Insights to Everyone with IBM Cognos Analytics 11